To integrate Jira with Abacus.AI, you can either use api token or directly login to your Jira account.
- Visit https://id.atlassian.com/manage-profile/security/api-tokens and generate a new API token. Be sure to copy and save it.
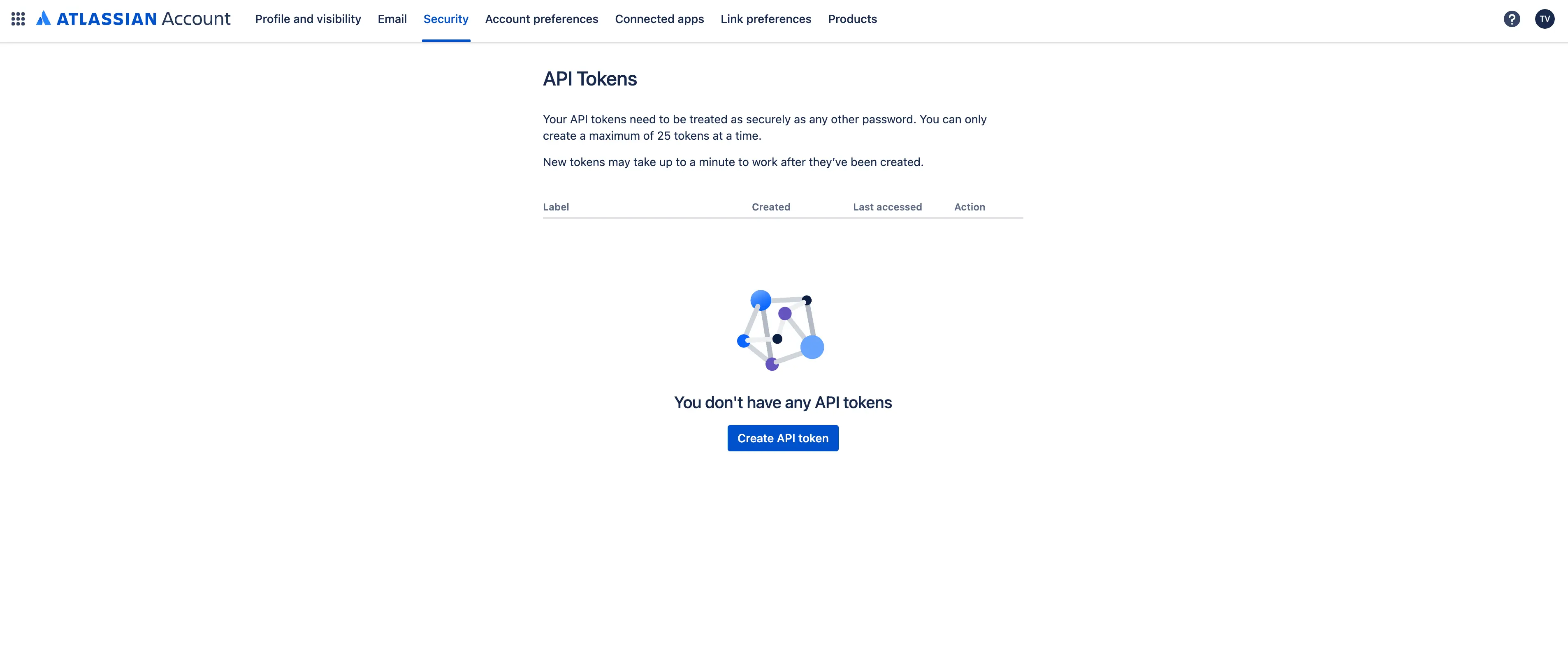
- In the Abacus.AI interface, click on your profile picture in the top right corner.
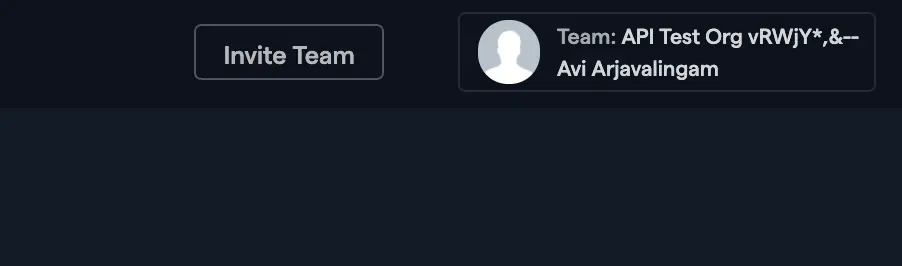
- Select "Manage Connectors" from the dropdown menu.
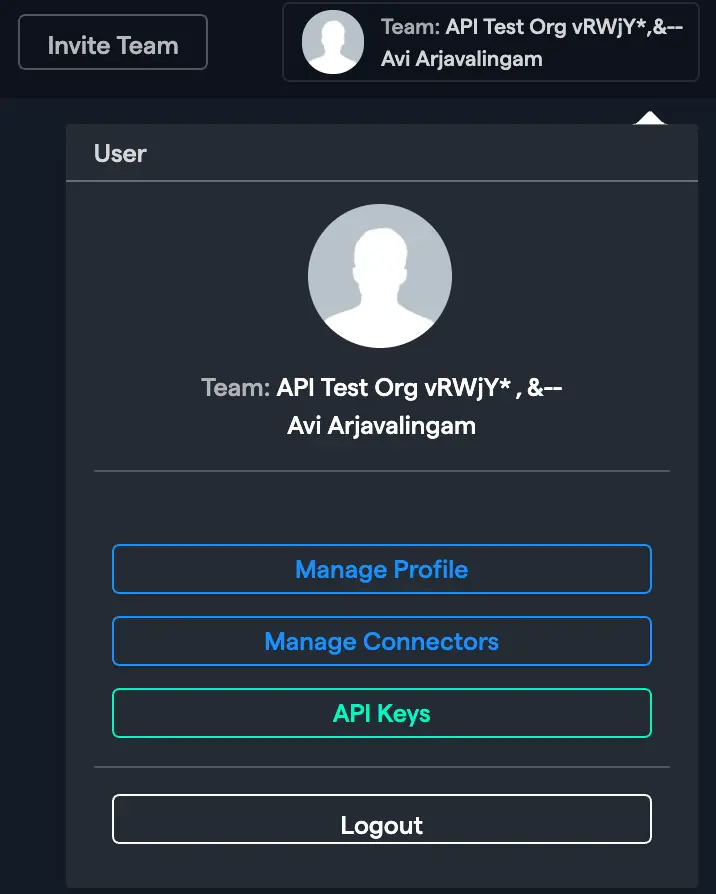
- Click on "Add New Connector", choose "Jira" from the list. Enter the name of the connector and enter the required information including the API token you generated.
Click "Save" to complete the setup.
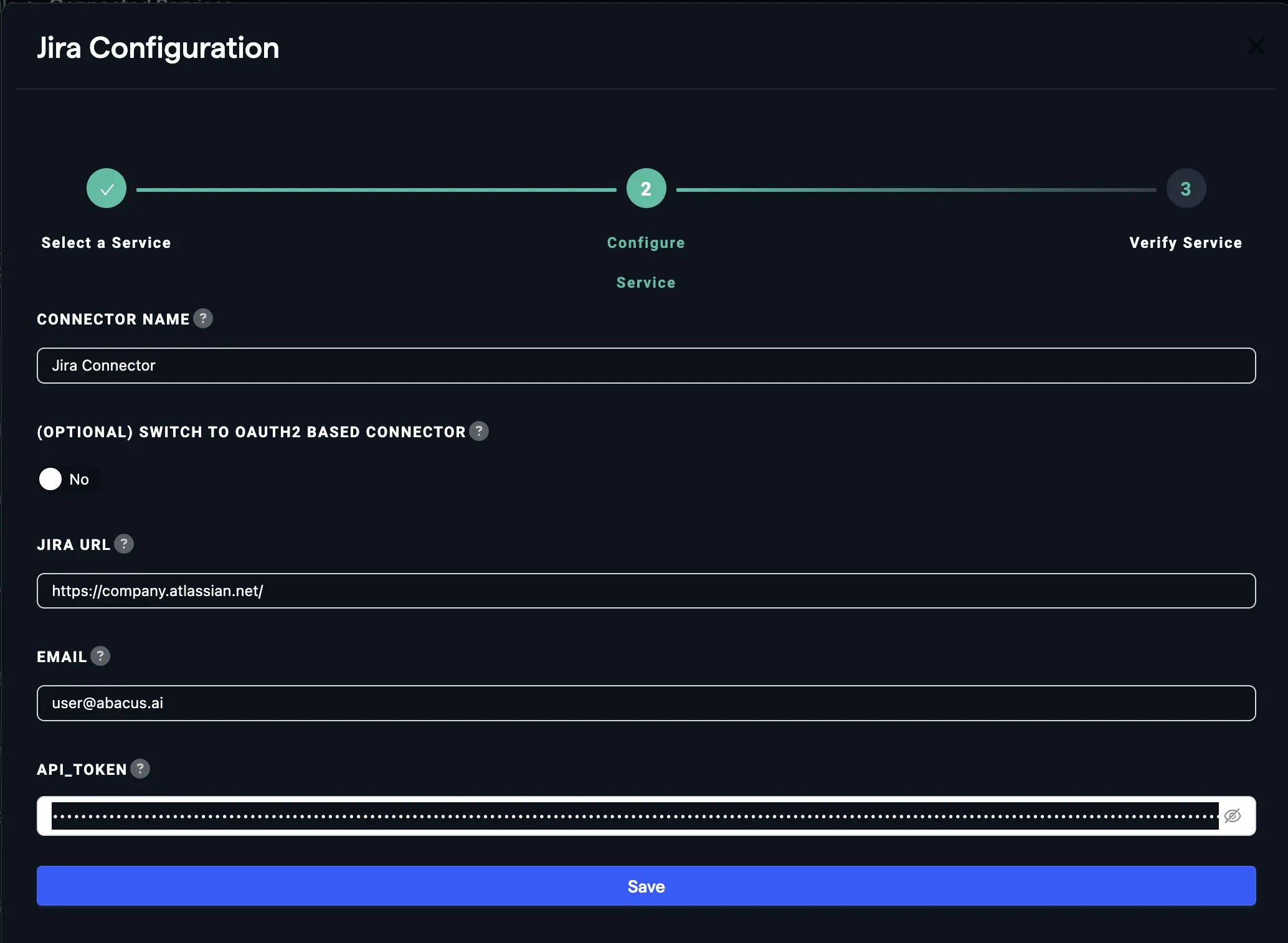
You can also directly login to your Jira account if you select the "switch to oauth based connector" option.
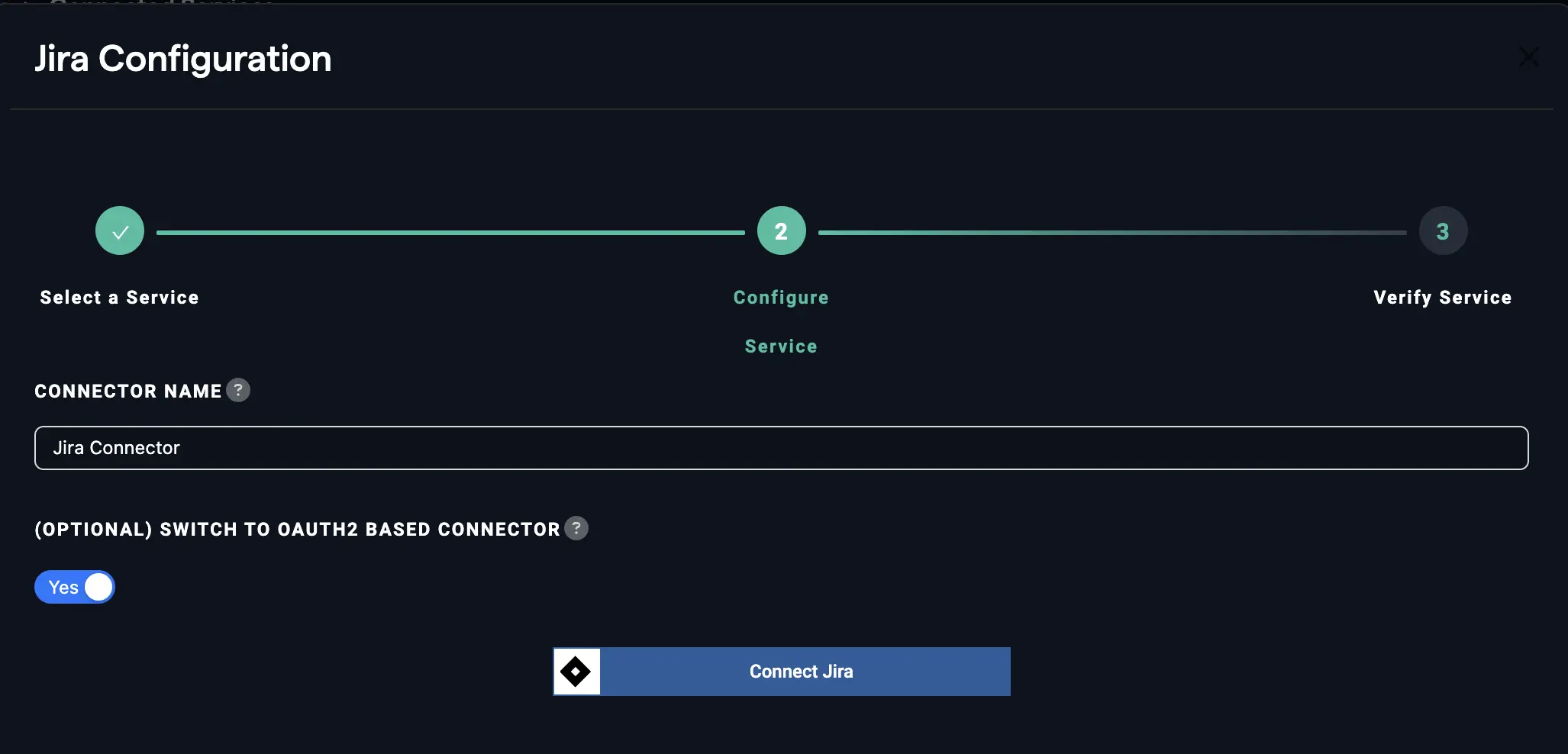
Once the Jira connector is set up, you can fetch data to train models in Abacus.AI.
- Create a new project and select the use case, then go to the "Datasets" tab and click "Create Dataset".
- Click on "Create New" and name the dataset.
- Choose "Read from External Service" and select your Jira connector under "Application Connectors".
-
Configure the specific data you wish to import from Jira using the special options provided:
- JQL Query: Enter a JQL query to filter the issues you want to include in your dataset. For help with JQL, visit this Atlassian JQL overview.
- Custom Fields: Select which custom fields you want to include in your dataset.
- Include Comments: Check this option if you want to include comments from the issues.
- Include Watchers: Check this option if you want to include information about who's watching the issues.
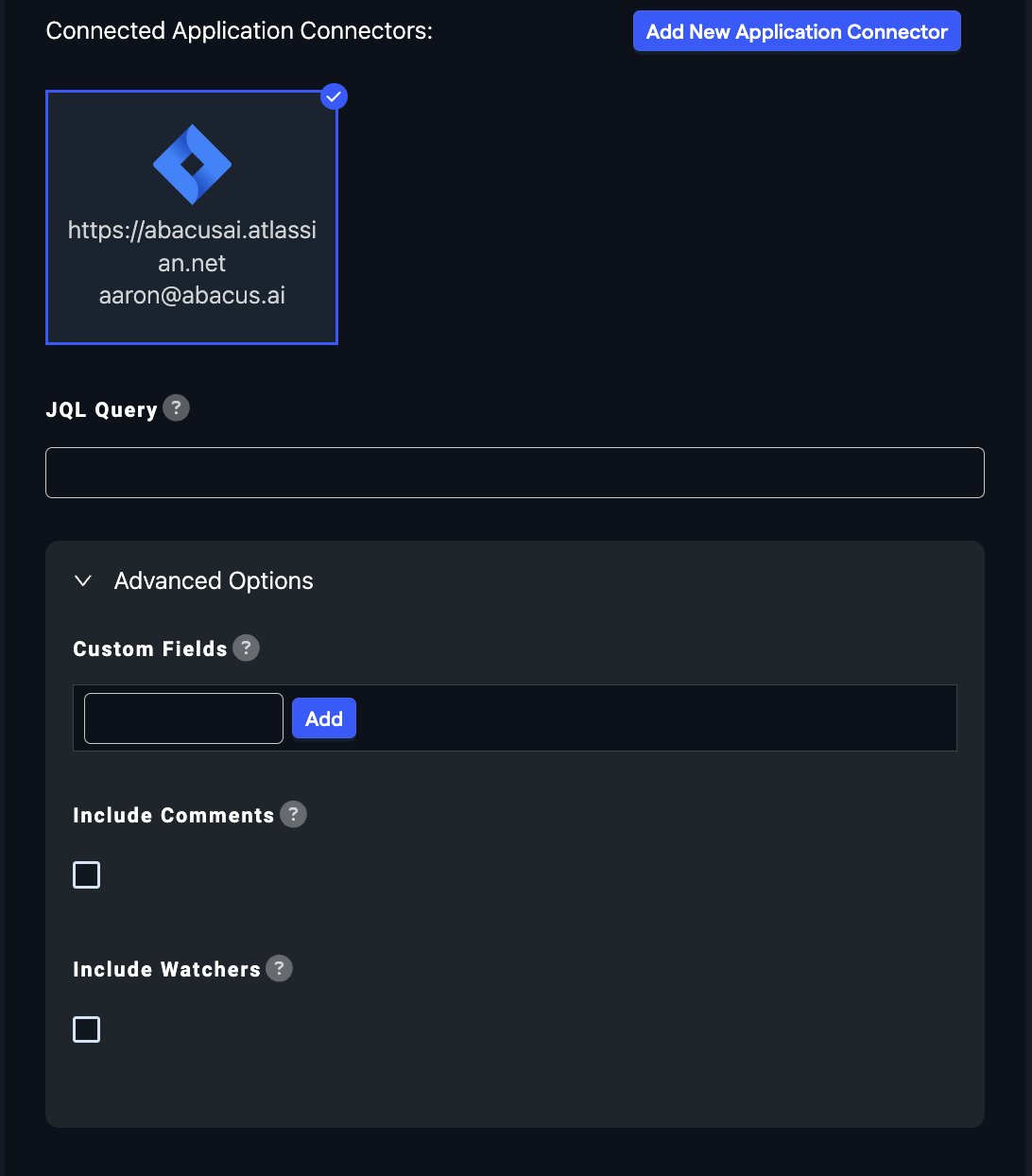
- After configuring the data import options, proceed to upload the dataset.
- Once the dataset is uploaded, configure the schema mapping and train models with the data.
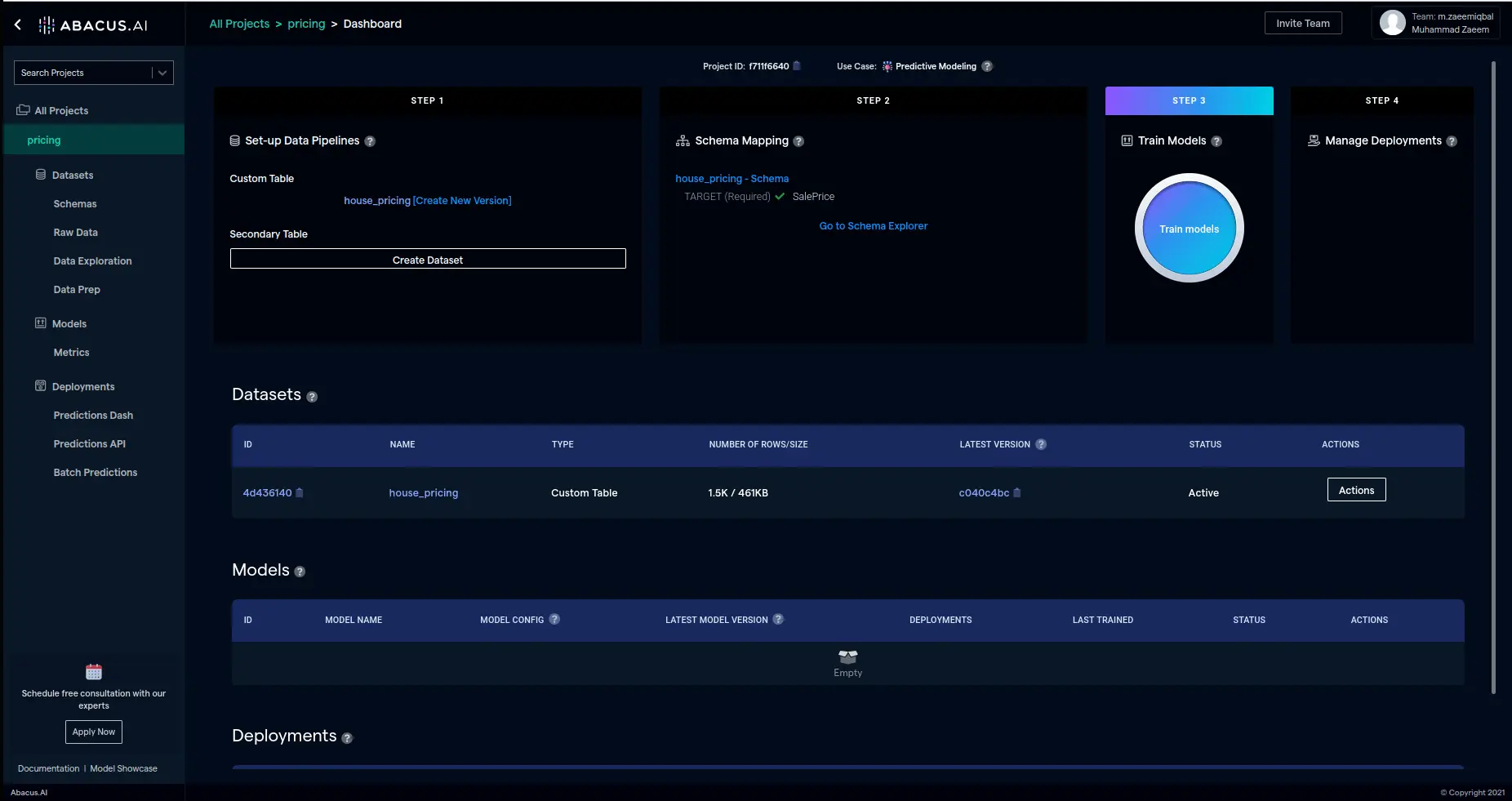
Realtime synced datasets are a feature in Abacus.AI that allows for continuous, automatic updates of data from external sources into the platform. This ensures that the data in your Abacus.AI projects is always up-to-date with the latest information from your connected systems.
** Note ** One must use Jira Admin credentials to set up a realtime synced dataset for Jira.
-
Connection to External Sources: Abacus.AI can establish connections with various external data sources, including project management tools like Jira and communication platforms like Microsoft Teams.
-
Automatic Synchronization: Once connected, the platform continuously monitors these sources for changes and updates. When new data is available, it's automatically synced to your Abacus.AI project.
-
Data Freshness: This ensures that your models and analyses are always working with the most current data, which is crucial for accurate predictions and insights.
-
Reduced Manual Work: It eliminates the need for manual data uploads or periodic batch updates, saving time and reducing the risk of human error.
- Issue Tracking: Abacus.AI can sync data about Jira issues in real-time. This includes information like issue status, assignees, priority, and custom fields.
- Project Management: Data about Jira projects, sprints, and epics can be continuously updated in Abacus.AI.
-
Use Cases: This data can be used for various predictive modeling tasks such as:
- Predicting issue resolution times
- Forecasting project completion dates
- Identifying potential bottlenecks in workflows
- Analyzing team performance and capacity
- Connect the Data Source: Set up the connection between Abacus.AI and your Jira or Teams instance.
- Configure Sync Settings: Specify which data should be synced and how often.
- Create Feature Groups: The synced data will be available as Feature Groups in your Abacus.AI project.
- Use in Models: Incorporate this continuously updated data into your machine learning models or analyses.
By leveraging real-time synced datasets, you can ensure that your data-driven decisions are based on the most current and accurate information available.